Secure ML: Automated Security Best Practices in Machine Learning
Alejandro Saucedo
As data science capabilities scale, the core concept of security becomes growingly critical. In this talk we will introduce the security challenges that data science practitioners face across the different phases of the machine learning lifecycle, including experimentation, productionisation and monitoring. We will also cover the set of frameworks and best practices that can be used to mitigate these security challenges at each relevant phase of the machine learning lifecycle. We will use a practical example that will allow data science practitioners to adopt these best practices in their daily workflows to ensure a relevant level of security is present in the multiple stages of the machine learning lifecycle.
Alejandro Saucedo
Affiliation: The Institute for Ethical AI & Machine Learning
Alejandro Saucedo is Director of Engineering at Seldon Technologies, where he leads teams of machine learning engineers focused on the scalability and extensibility of machine learning deployment and monitoring products. Alejandro is also the Chief Scientist at the Institute for Ethical AI & Machine Learning, where he contributes to policy and industry standards on the responsible design, development and operation of AI, including the fields of explainability, GPU acceleration, privacy preserving ML and other key machine learning research areas. With over 10 years of software development experience, Alejandro has held technical leadership positions across hyper-growth scale-ups and has a strong track record building cross-functional teams of software engineers. He is currently appointed as governing council Member-at-Large at the Association for Computing Machinery, and is currently the Chairperson of the GPU Acceleration Kompute Committee at the Linux Foundation.
LInkedin: https://linkedin.com/in/axsaucedo Twitter: https://twitter.com/axsaucedo Github: https://github.com/axsaucedo Website: https://ethical.institute/
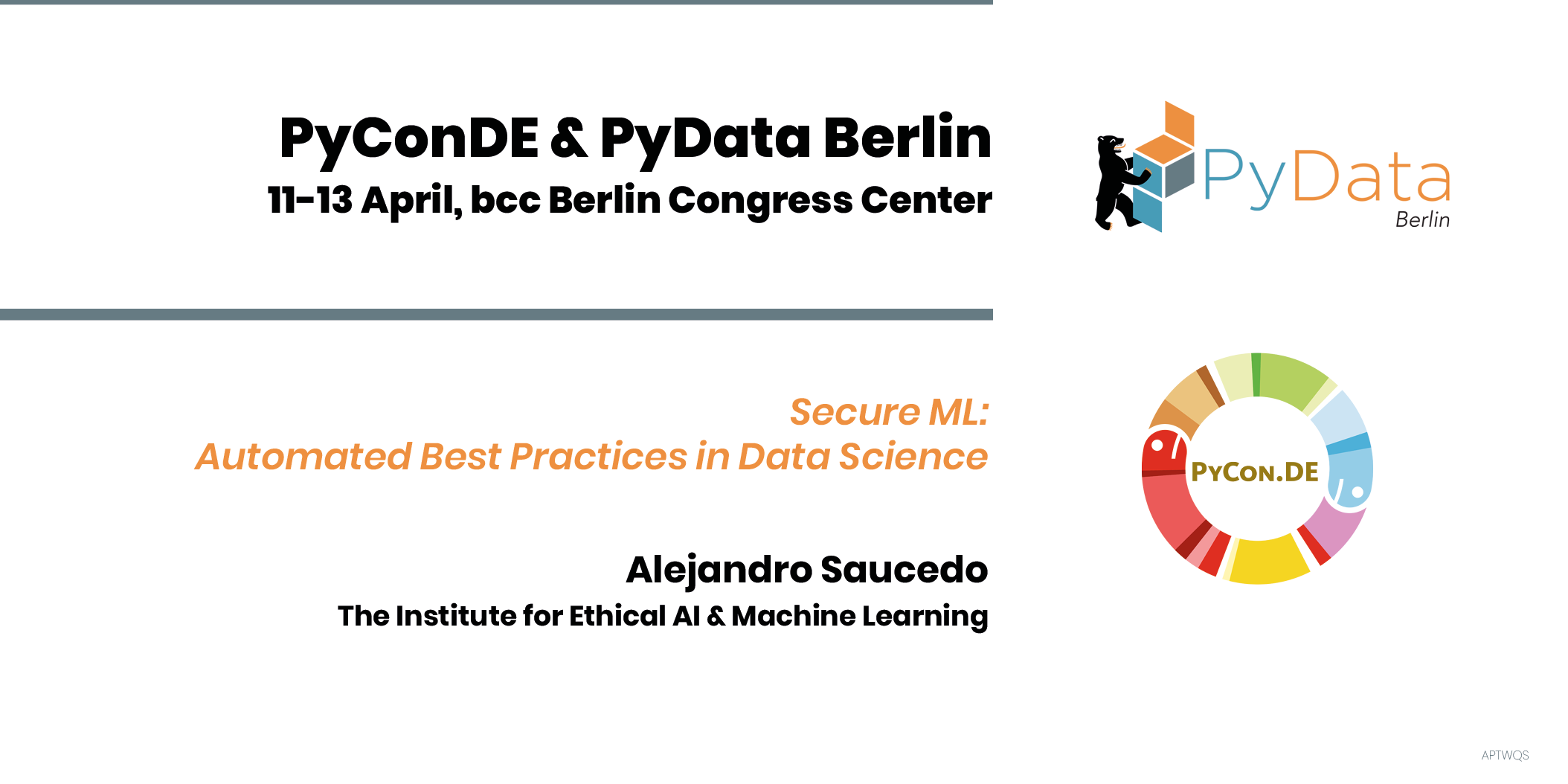