Detecting drift: how to evaluate and explore data drift in machine learning systems
Emeli Dral
When your ML model is in production, you might observe data and prediction drift: a meaningful change in the input data distributions and model output. In cases where you don't get the true labels or actual values fast, this drift might be the only proxy for the model performance. Drift analysis can also be helpful in debugging the model performance drop. But how exactly to evaluate it in practice? Should you look at descriptive feature statistics, apply statistical tests to compare distributions, and which exactly? In this talk, I will give an overview of the possible approaches to drift detection, and how to implement and visualize the results.
Emeli Dral
Affiliation: Evidently AI
Emeli Dral is a Co-founder and CTO at Evidently AI, a startup developing open-source tools to analyze and monitor the performance of machine learning models.
Earlier, she co-founded an industrial AI startup and served as the Chief Data Scientist at Yandex Data Factory. She led over 50 applied ML projects for various industries - from banking to manufacturing. Emeli is a data science lecturer at GSOM SpBU and Harbour.Space University. She is a co-author of the Machine Learning and Data Analysis curriculum at Coursera with over 100,000 students. She also co-founded Data Mining in Action, the largest open data science course in Russia.
visit the speaker at: Github
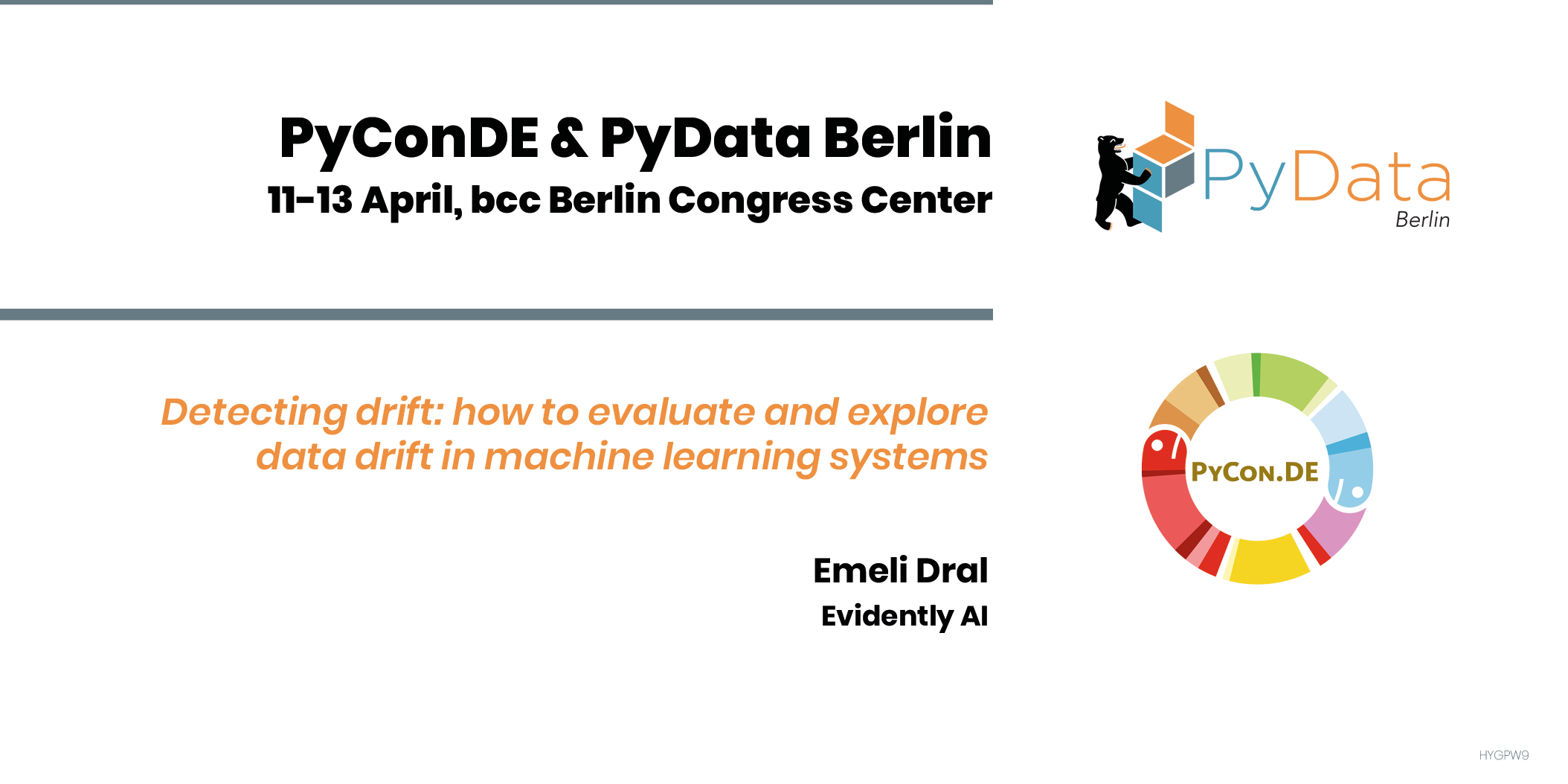