Introduction to MLOps with MLflow
Tobias Sterbak
Machine learning requires experimenting with different datasets, data preparation steps, and algorithms to build a model that maximizes some target metric. Once you have built a model, you also need to deploy it to a production system, monitor its performance, and continuously retrain it on new data and compare with alternative models. A possible solution to managing parts of this complexity is offered by MLFlow.
Please make sure to follow the instructions on https://github.com/tsterbak/pydataberlin-2022 to setup your python environment before attending! That way everything will run smoothly :)
In this tutorial, you will learn how to use MLflow to:
- Set up a tracking server and a model repository.
- Keep track of machine learning training and experiment results (parameters, metrics and artifacts) with MLflow Tracking.
- Package the training code in a reusable and reproducible format with MLFlow Projects.
- Deploy the model into a HTTP server with MLFlow Models and keep track of it's state.
Tobias Sterbak
Tobias Sterbak is a Data Scientist and Software Developer from Berlin. He has been working as a freelancer in the field of Natural Language Processing since 2018. On the blog www.depends-on-the-definition.com he occasionally writes about topics in Machine Learning and Natural Language Processing. In his private life he is interested in data privacy, open source software, remote work and dogs.
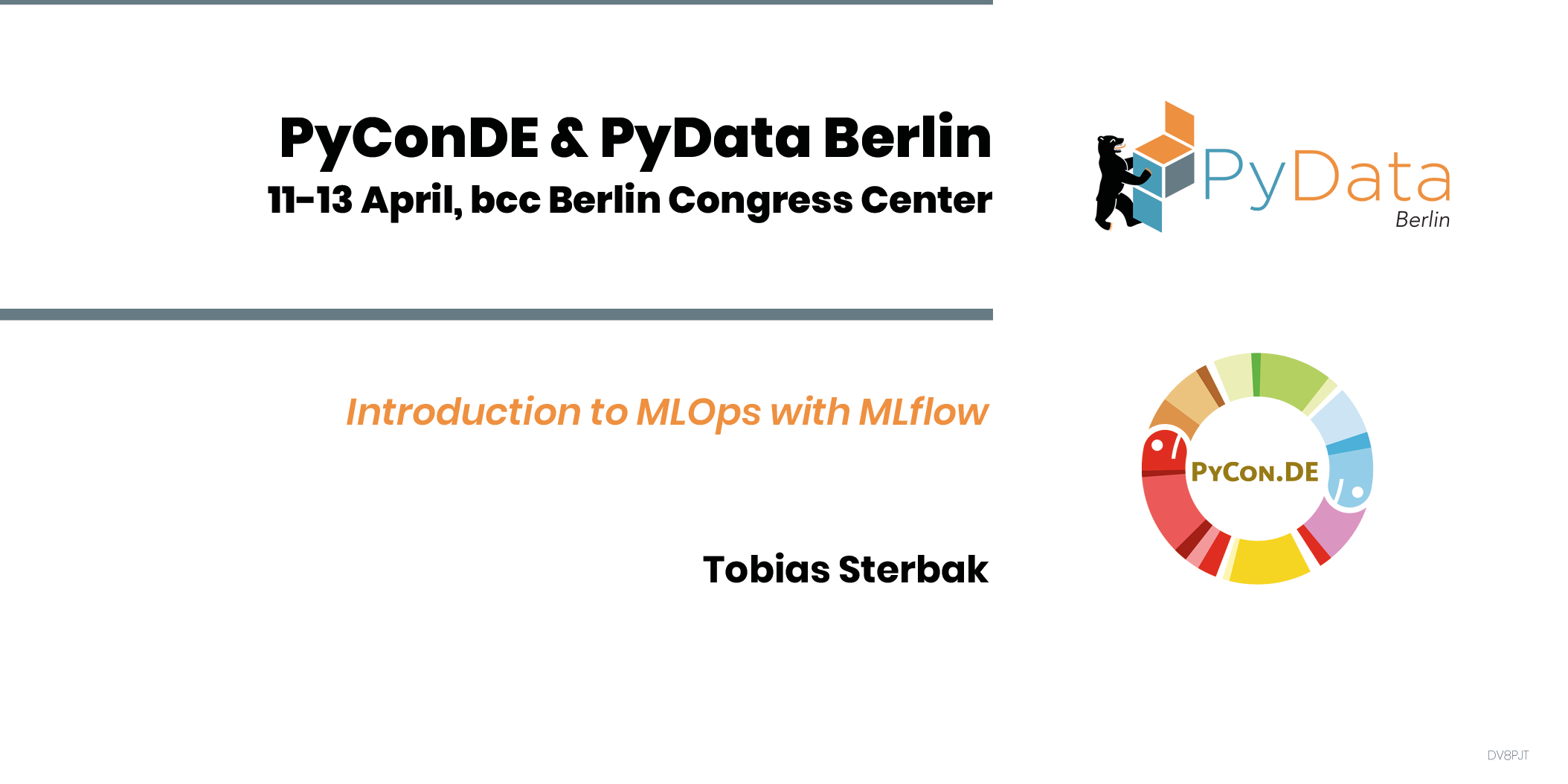