Introduction to Uplift Modeling
Dr. Juan Orduz
In this talk we introduce uplift modeling, a method to estimate causal effects of a treatment, e.g. a marketing campaign, to effectively target customers that are most likely to respond to it. We describe the most common methods to estimate such effects by working a concrete example. Specifically, meta-algorithms to estimate conditional average treatment effect (CATE) using machine learning estimators.
References:
- Pierre Gutierrez and Jean-Yves Gerardy. Causal inference and uplift modeling a review of the literature. JMLR: Workshop and Conference Proceedings 67, 2016.
- https://causalml.readthedocs.io/en/latest/methodology.html
- https://www.uplift-modeling.com/en/latest/user_guide/index.html
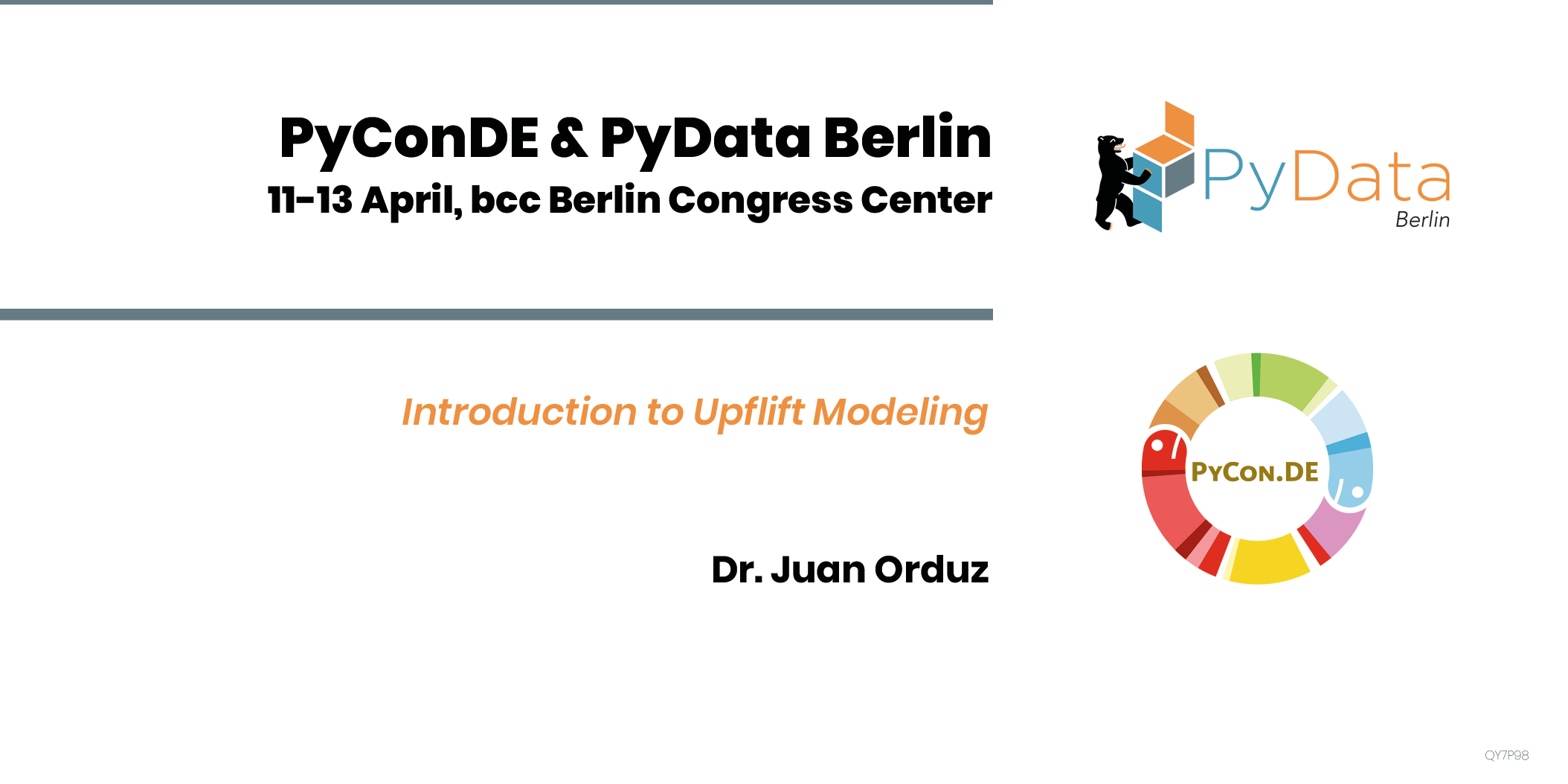