My forecast is better than yours! What does that even mean?
Illia Babounikau
Forecasting is one of the most popular applications of Machine Learning. In the last decades, it went from large numbers, few factors, and simple algorithms to small numbers, many factors, and complex ML models. Moreover, some modern forecasting models can predict not only naive point estimators of the target variable but their probability distributions. As an example, BlueYonder delivers demand forecasts in the form of demand probability distribution on a very granular level (e.g. for each product, store, and day).
However, established forecast evaluation procedures and criteria (e.g. directly using metrics like RMAE, RMSE, MAPE, etc., and comparing these metrics between various data categories) often turn out to be inappropriate and biased. Therefore, it is important to understand the limitations of the traditionally used metrics and approaches. BlueYonder has implemented forecast evaluation techniques to address these limitations.
In this talk, I will present the number of forecast evaluations issues and possible resolutions:
Main pitfalls when evaluating time series forecast for counted values. Be careful! An inappropriate metric can lead to wrong conclusions and selecting worse model!
Fundamental limitations of forecast accuracy. It could be impossible to improve forecast quality. Know your limits!
How to correctly compare forecast quality for different data subsets or different forecasting algorithms. Apple-to-apple comparison is not as easy as we think.
All of the above points will be covered based on the real use cases of demand forecasting developed within BlueYonder.
Illia Babounikau
Affiliation: BlueYonder
I have finished PhD in particle physics in 2019 at CERN and DESY. It was fun to search for new fundamental particles with advanced ML techniques. Since 2020, I have been enjoying supporting customers with BlueYonder ML solutions, developing internal tools for forecast evaluation and onboarding new data scientists.
visit the speaker at: Homepage
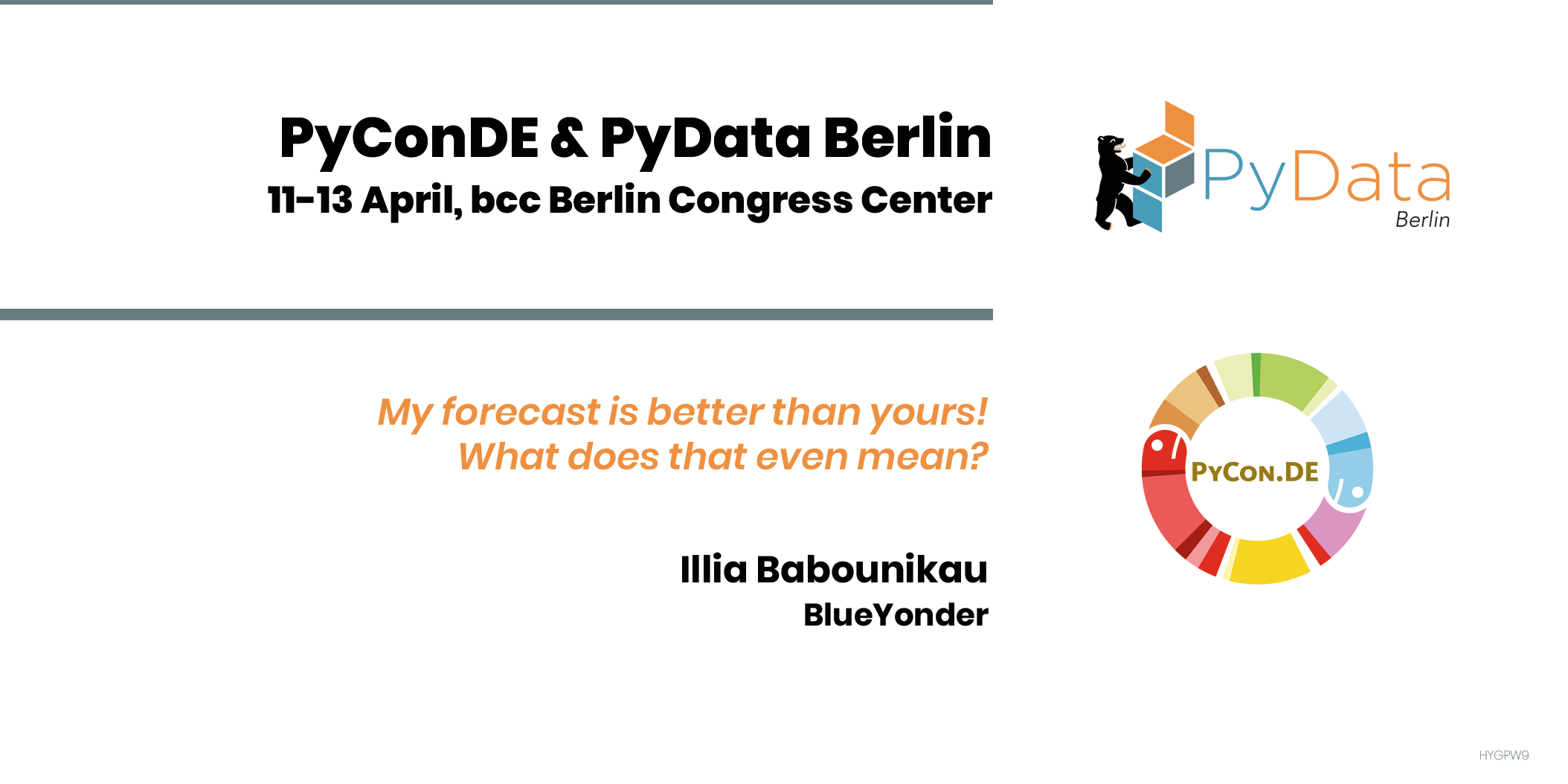