Performing Content: Can NLP and Deep Learning algorithms predict reader preferences?
Sebastian Cattes
User engagement is one of the most important factors to steer and optimize the content delivered to customers, e.g. readers of online newspapers. Being able to predict this KPI for new, not yet published articles is a key factor in process optimization and state-of-the-art tools to assist editors in their daily work flow.
The talk shows that standard onsite tracking KPIs can be used to reveal driving mechanisms to model and predict reading times for articles published online. It will be discussed how statistical (GAM) and NLP related deep learning algorithms (Transfer Learning using BERT) can be combined to distinguish different driving mechanisms. Further insight about text characteristics and content that increases reader engagement is derived by applying Explainable AI (XAI) techniques in combination with less transparent machine learning approaches (XBG).
Sebastian Cattes
Affiliation: INWT Statistics
Sebastian Cattes works as a Data Scientist for INWT Statistics, a Berlin based statistics and data science consulting company.
After joining INWT’s marketing analytics department in 2018 he has worked on a variety of projects such as online marketing, sustainable investment strategies and predictive maintenance.
He is specialized in statistical analysis of time series data, technical development and deployment of data science products in Python and R, as well as Natural Language Processing. The use of unstructured text data is getting more and more important in data science projects, causing him to focus on modern Deep Learning techniques.
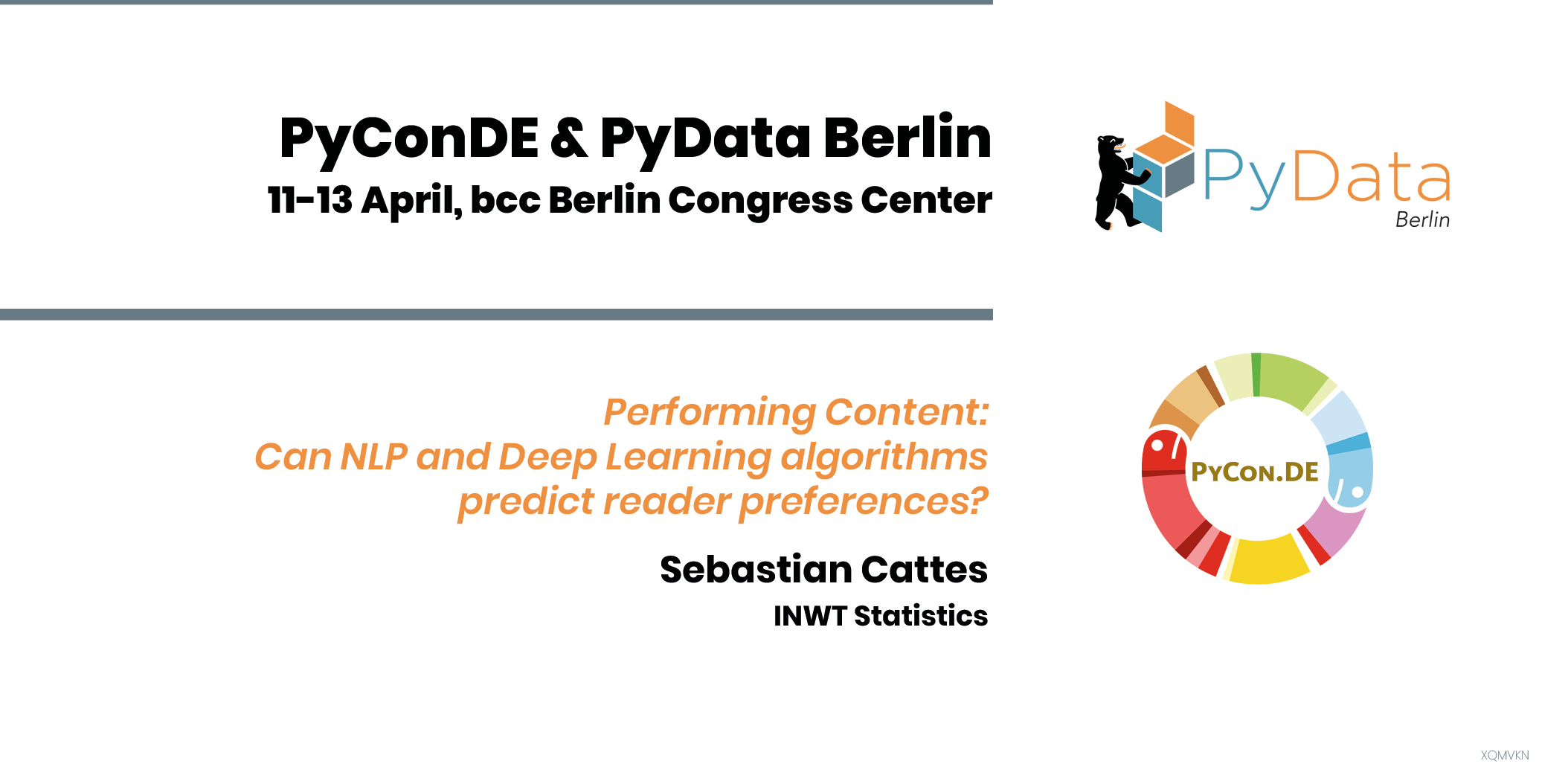